On an average, we make about 70 conscious decisions in a typical day. Our brain makes dozens of micro decisions (boolean decisions) every single second. Choices, sub-choices, super-sub-choices, tiny little micro decisions building up decision trees and forests to arrive at a macro decision. It takes seconds sometimes and sometimes even days to years in arriving at one. We cannot get by our daily lives without deciding on something. Decision making is an integral part of our life, some even consider it an art and others call it a proficiency. Whether it is an art or a proficiency, one thing is certain, there is always someone at the center of it, the decision maker. Whether you believe in the simulation hypothesis or in the law of attraction, It’s all about the person at the center, the decision maker, a human, an agent or an automated system (an entity), who picks a particular choice from the available options.
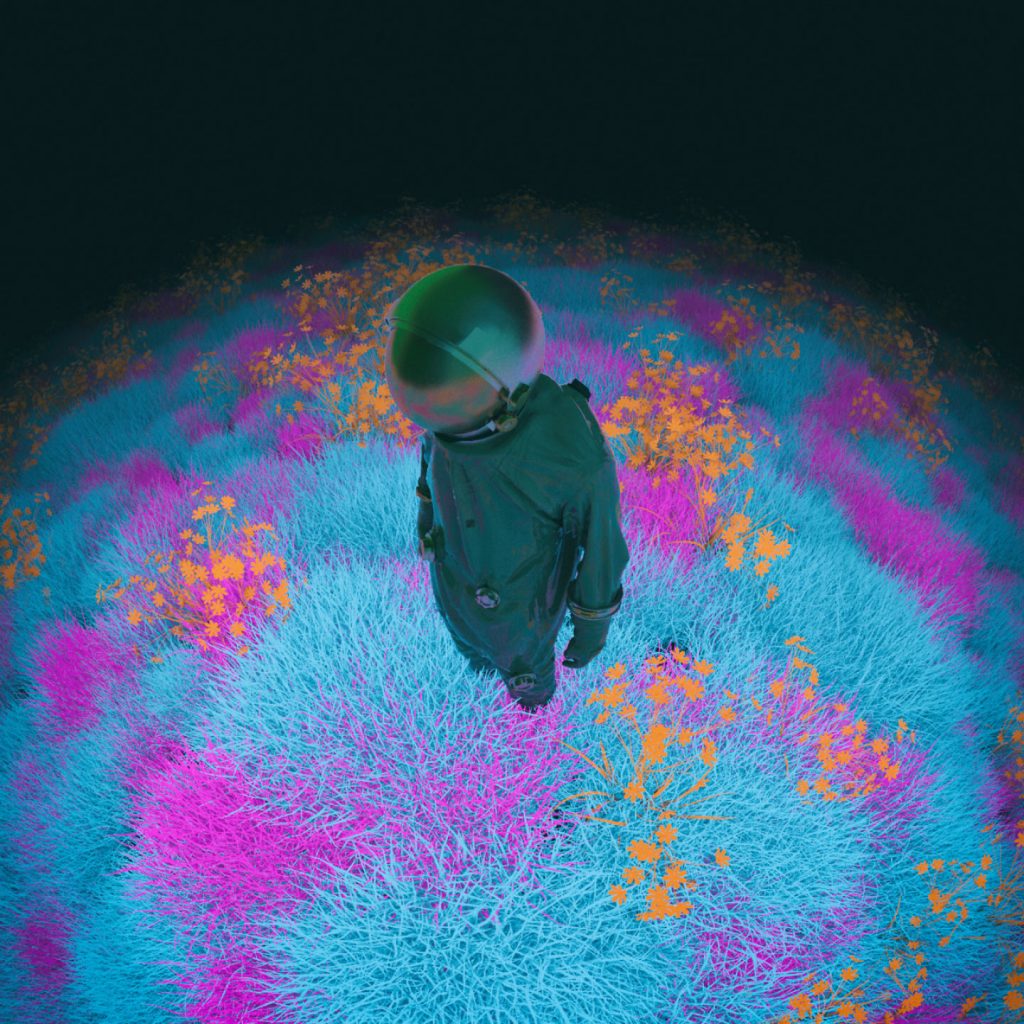
The Decision Maker & The Best Choice
The decision maker or the entity is deemed “intelligent” or “smart” if there’s an underlying criterion or rationale behind picking a particular choice. The degree of intelligence or smartness is determined by the ability to pick the “best” choice from the available options (obviously, the entity or the selection is not considered intelligent if the choice is random). Now, what does this underlying criteria or rationale function on? IMO, there are three core aspects that are absolutely essential in picking the best choice, first, ‘available information’, which helps in identifying, eliminating and narrowing the options (sometimes, the noise from the observations increases the effort to eliminate options, which results in poor quality of decisions). Second, the ‘time’, the total available time to make the decision which provides a sense of urgency or latency to the act. Finally, the ‘reward’, which mostly is the primary motivation for making the decision. For the purpose of this article, let’s focus on the first part of this rationale, the ‘available information’.
The Seeker, Available Information & The Giver
How do we gather the required information before picking the best option? It depends on the situation, isn’t it? if it’s a repetitive situation then, we depend on a previously built mental model, in other words ‘experience’. If it’s a new situation then we build newer models with available information and depend on the experience model for future occurrences. We have a tendency to depend on our personal preferences for simpler choices but, when it comes to complex decisions, we tend to gather more information. One of the quick hacks our brain employs in solving a complex decision problem is depending on an expert’s recommendation. Why not? We are social beings, why not use social information (especially when we face a lack of necessary information sitch) to solve a troubling decision problem. But wait, how do we filter these social recommendations? This is where “trust” comes into the picture. Trust, a dependable model our brain builds over a period of time, based on past experiences to hack a complex decision-making task. Now, for certain situations, the entire process of decision making boils down to the binary relationship between ‘the seeker’ (the decision maker) and ‘the giver’ (the expert who gives the recommendation).
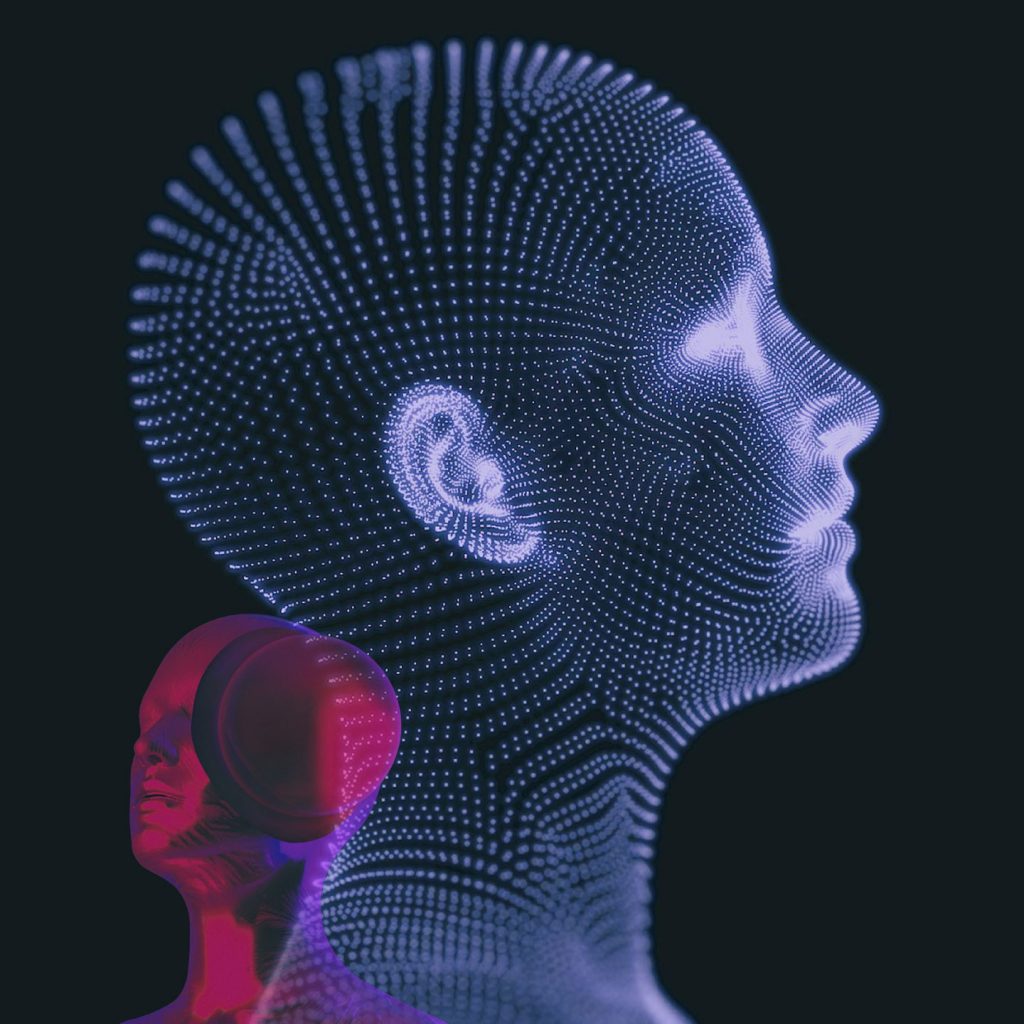
Core Attributes Of An Expert
What are the core attributes of an “expert” who can give us the ‘best’ recommendation? Being “expert” at the subject is certainly an important criteria, we have also established “trust” to be a qualifying factor in our mental model, “accessibility” is another attribute that can directly appeal to the time component we have talked about earlier and yes, should be “intelligent” to understand the seeker and the “context” to give the most “relevant” and “accurate” recommendation to qualify it as the “best” recommendation from the available options.
What Happens In The Real-World?
Let’s understand this model from a trade and business context. When you enter a store, a real store (offline store with walls, furniture, merchandise, and salespeople), salespeople act or approach as the experts in a store to guide us in our decision-making process, that is their role, they often receive training on the sales process, they have knowledge on business profit numbers and they are trained to respond to our queries about the product and to help us make the best choice. From a business perspective, salespeople are not scalable, nor economically viable so, businesses invented a non-technical hack called POGs (‘Plan-o-grams’ or ‘Planograms’) to arrange items to increase visibility on high-profit and high-frequency items to increase revenues and optimize the store spaces. Some online stores borrowed the same idea to display their high-profit, high-frequency items on their homepage layouts to optimize their sales. The problem with online stores is the absence of a definitive sales guide and population of their online stores with the abundance of options. Sometimes, there are too many options and too many choices available and users do not like to invest their time in going through the entire catalog to find the best choice. An obvious answer to this problem is an intelligent recommendation system, a system that can mimic the role of a salesperson, a system that can reduce the workload on users who are overwhelmed by the number of available options. Not just help in reducing options; typically, in a customer journey, customers go through stages like need, awareness, research, comparison, decision & purchase. Other than the initial need and final purchase stage, an intelligent recommendation system should be able to assist customers through the stages of awareness, research, and decision.
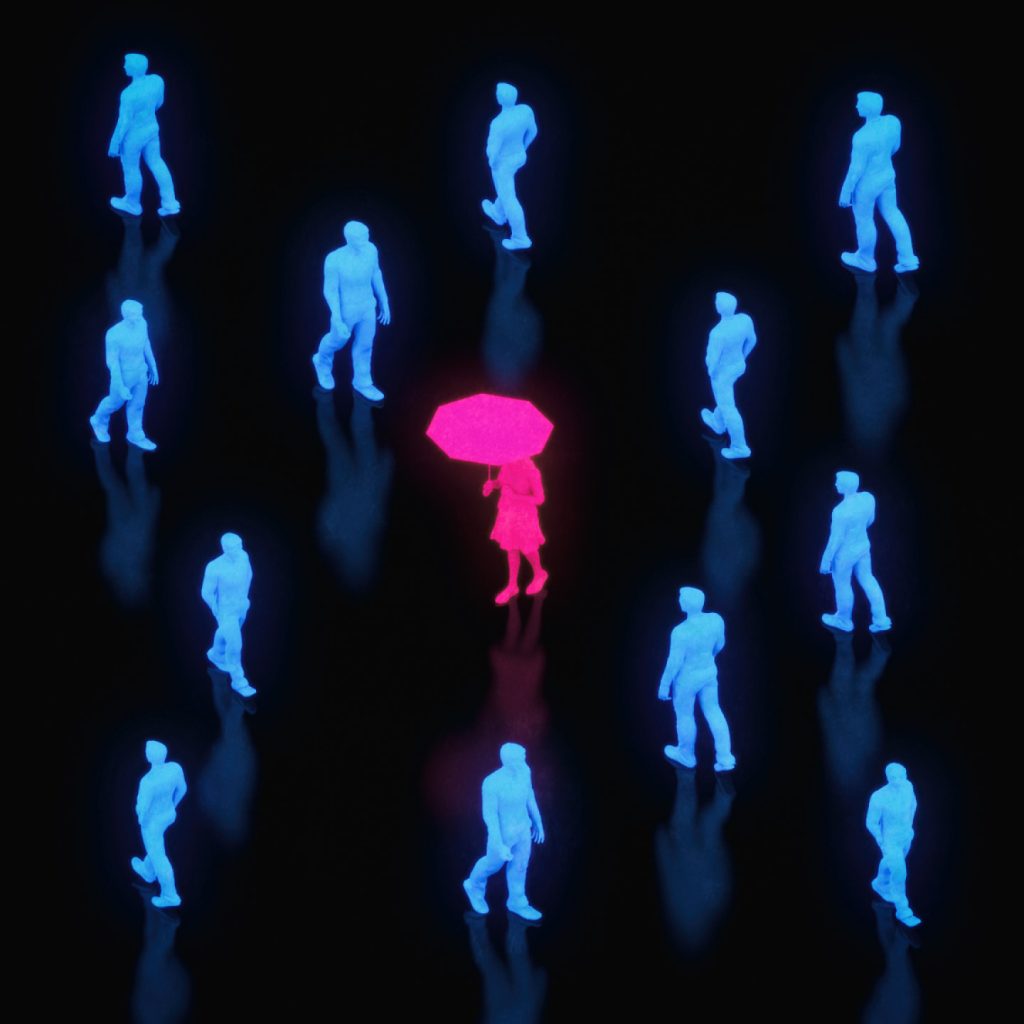
Every Successful Product Or Business Has A Strong Recommendation Engine At Its Core.
Amazon’s — “Customers who bought this item also bought…”.
Netflix’s — “Other Movies You May Enjoy…”
Spotify’s — “Recommended songs…”
Google’s — “Visually Similar Images…”
YouTube’s — “Recommended Videos…”
Facebook’s — “People You May Know…”
LinkedIn’s — “Jobs You May Be Interested In…”
Coursera’s — “Recommended courses…” and
Waze’s — “Best Route…”
are all results of strong recommendation systems at the core of these businesses. The impact of these recommendation systems is immense from a business standpoint as well.
According to this McKinsey’s article, 35% of Amazon’s revenue and 75% of what people watch on Netflix comes from their recommendation engines.
Macro Components Of An Intelligent Recommendation Engine
Now, from the context of building a product or a service with a strong recommendation system in place; it may be easier to build a fast, automatic, heuristic based system but, how can we put the decision maker, the user in control? How can we build an intelligent recommendation ‘engine’ that can mimic the dynamic interactive human nature between the seeker and the giver? How can we build an engine to understand the context, capture the immediate feedback on the recommended items and also calculates the long-term benefits for you, like a friend? Before that, let’s understand one of the important macro-components of an intelligent recommendation system — filtering models. What is a model? According to this “Models Will Run The World” article by Steven A. Cohen and Matthew W. Granade:
“A model is a decision framework in which the logic is derived by algorithm from data, rather than explicitly programmed by a developer or implicitly conveyed via a person’s intuition. The output is a prediction on which a decision can be made. Once created, a model can learn from its successes and failures with speed and sophistication that humans usually cannot match.”
In short, models are the true source of power behind robust business systems and recommendation (filtering) models are one of the most popular research subjects for data scientists today. There are 5 types of recommendation filtering models that I’ve come across: (the word ‘filtering’ is used because, filtering is a fundamental common requirement when dealing with large sets of data, filtering helps in focussing on relevant data).
Types Of Filtering Models:
1. Popularity Filtering Model:
This is the most basic and simplest of all models, the recommendations are based on the number of views, likes, ratings or purchases. Most popular items on the platform (product) are recommended to all the users. The limitation of this model is that it is uni-dimensional, i.e., the recommendations stay the same for every user.
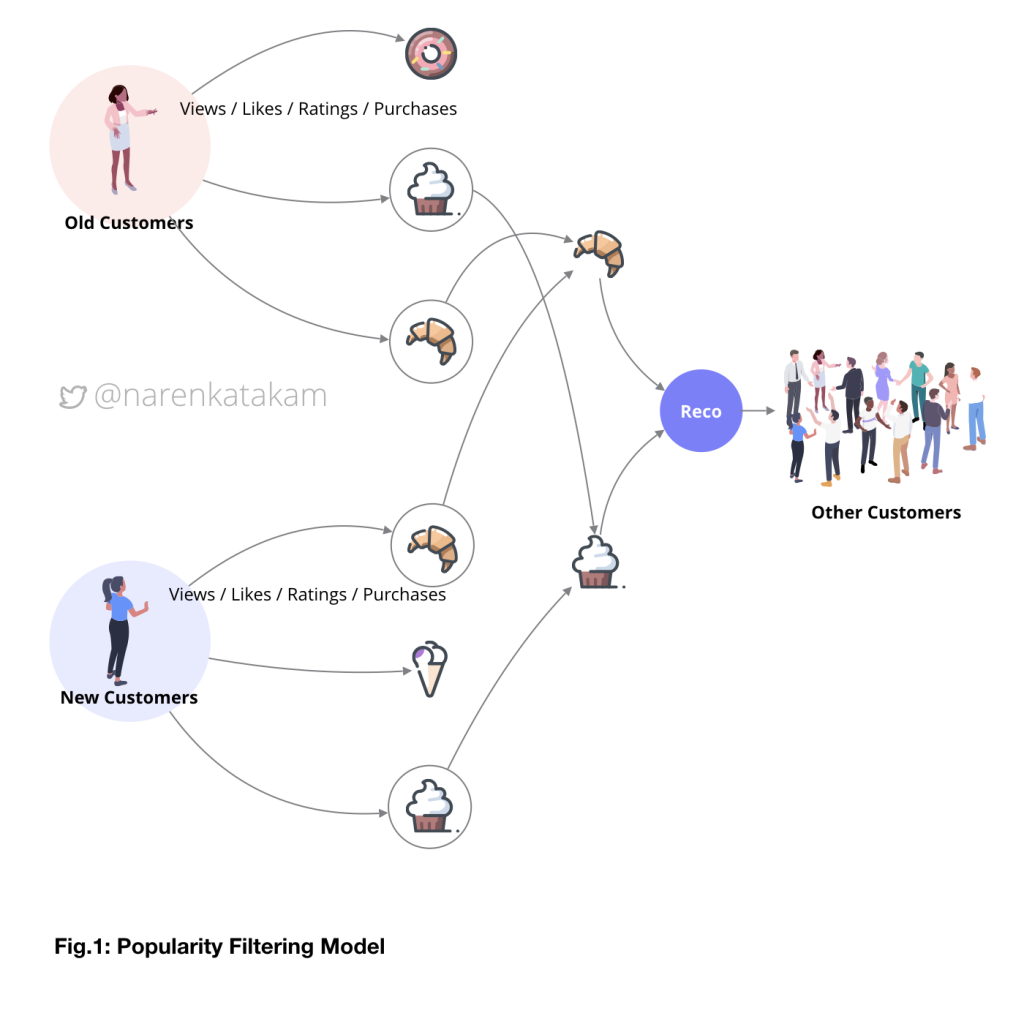
2. Collaborative Filtering Model:
This is a user-user collaborative model, it is more like an extension to the popularity model but, micro and more personalized. Information is collected on the users to find similar users and the recommendations flow based on the interactions of one user on the platform to another similar user. The limitation of this model is that the performance of the system slows down as the user base grows. Sometimes users are clustered into pairs and groups to speed things up. A trade-off product owners have to make with user personalization part in the favor of product speed.
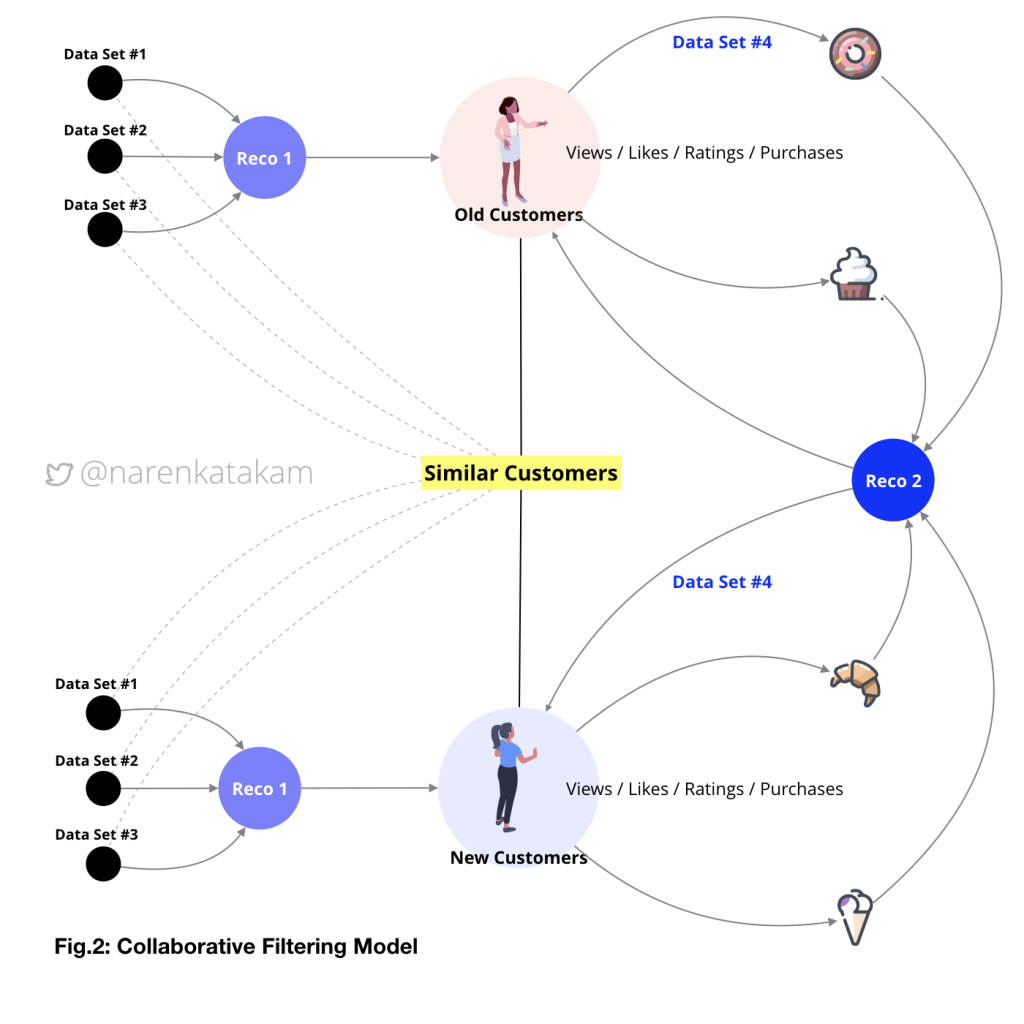
3. Content Filtering Model
The idea here is similar to the collaborative model except that the correlation is found between items and not users. That is the reason why this model is also known as the item-item collaborative model. However, it is extremely crucial for product owners to define a strong similarity framework. The advantage of this model is that the relationship between items can be more stable (depends on the similarity framework) than users and in general items are a smaller base compared to user base so, the speed of the system stays good even if the user base grows.
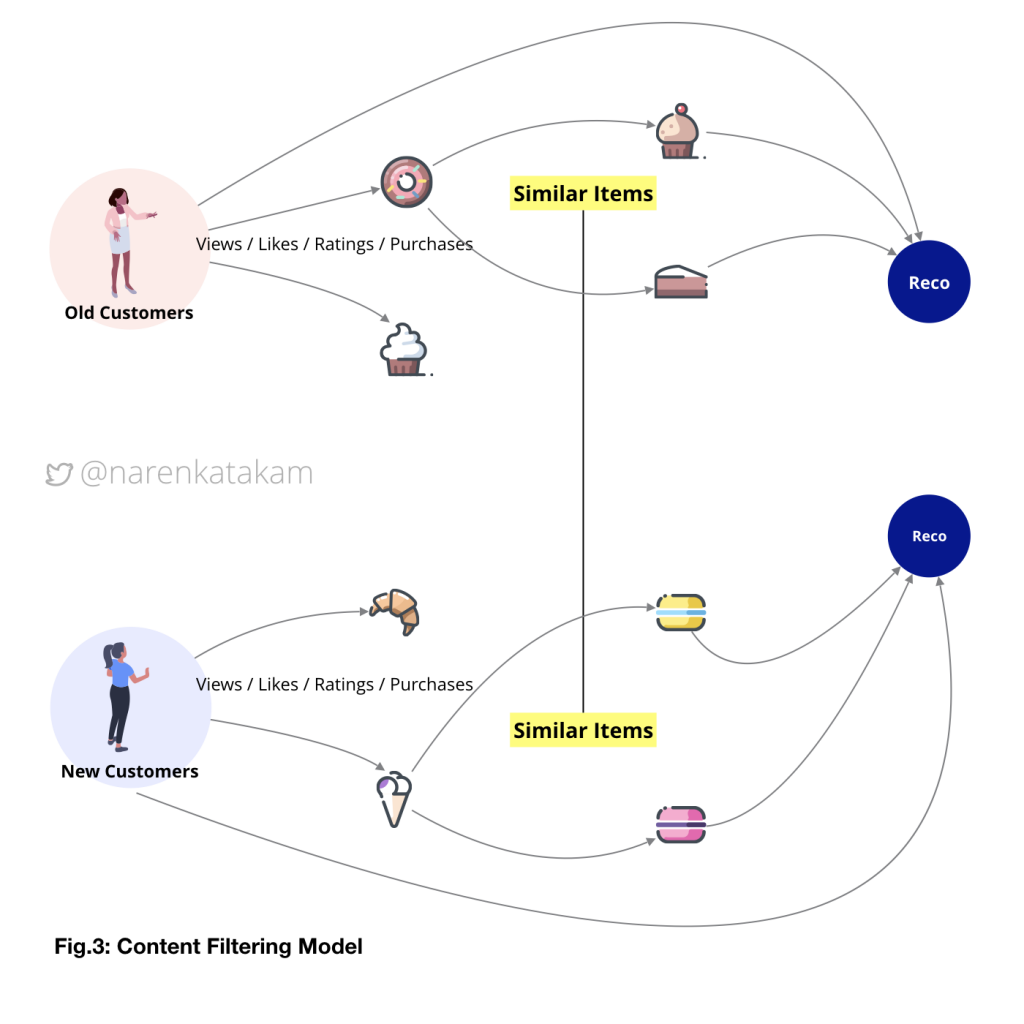
4. Association Rule Mining Model:
The idea here is an extension of the content filtering model. The difference is that the focus is shifted from the “items” to a “session”. What it means is, the items that appear together in the same ‘session’ are recommended to other users across the platform. For example, an association rule could be like: 80% of users who like item A and item B also like item C; and 20% of all users like all three items. Another rule could be like: 70% of all items liked by user X and user Y are also liked by user Z and 30% of all items are liked by all three users. The concept is based on association rules here, and the correlation is formed based on the ‘appearance’ in the same session. The limitation of this model is that it is less personalized compared to collaborative or even content filtering models.
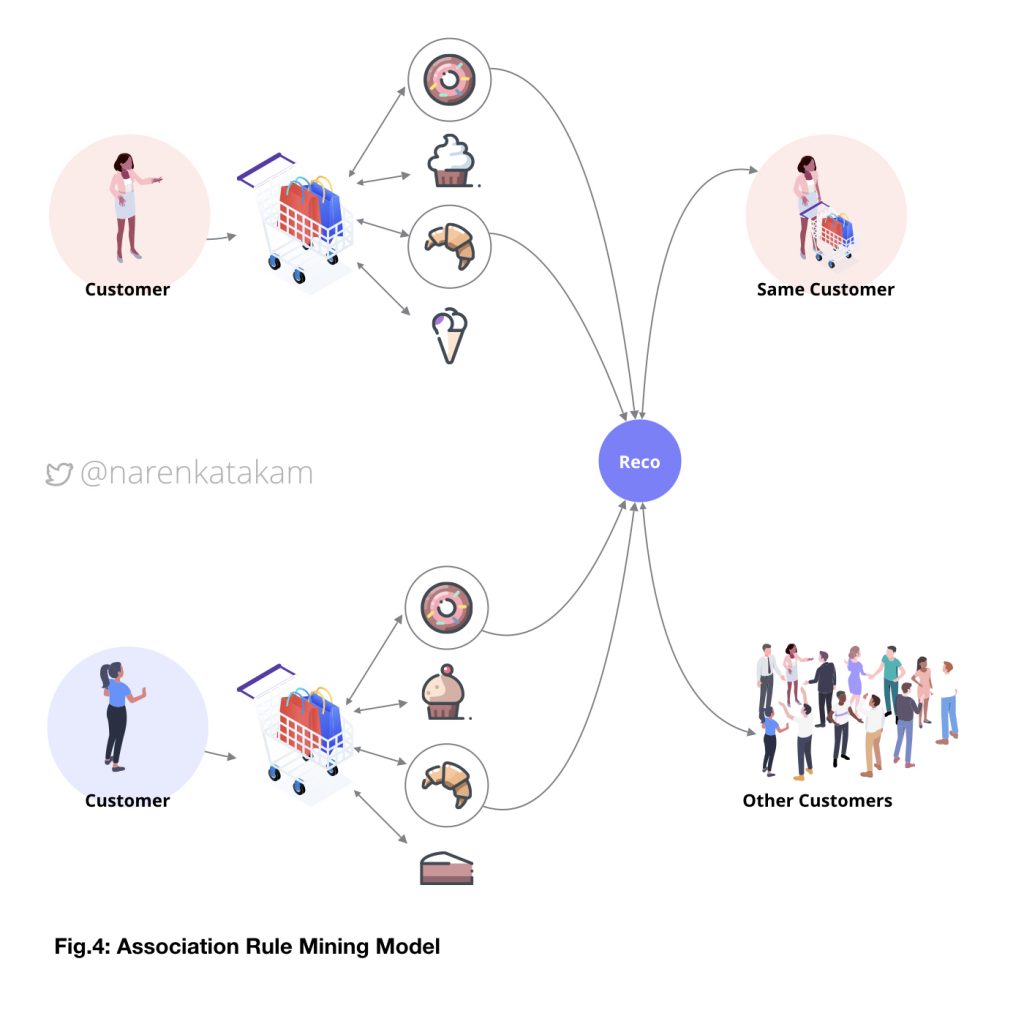
5. Hybrid Filtering Model
As the name suggests, this model combines two or more filtering techniques to achieve better performance. Usually, collaborative filtering methods are combined with content filtering methods in layered, weighted, switched, mixed and cascaded approaches to achieve desired results. The advantage of this model is that it is more powerful, more stable and more relevant compared to the other models.
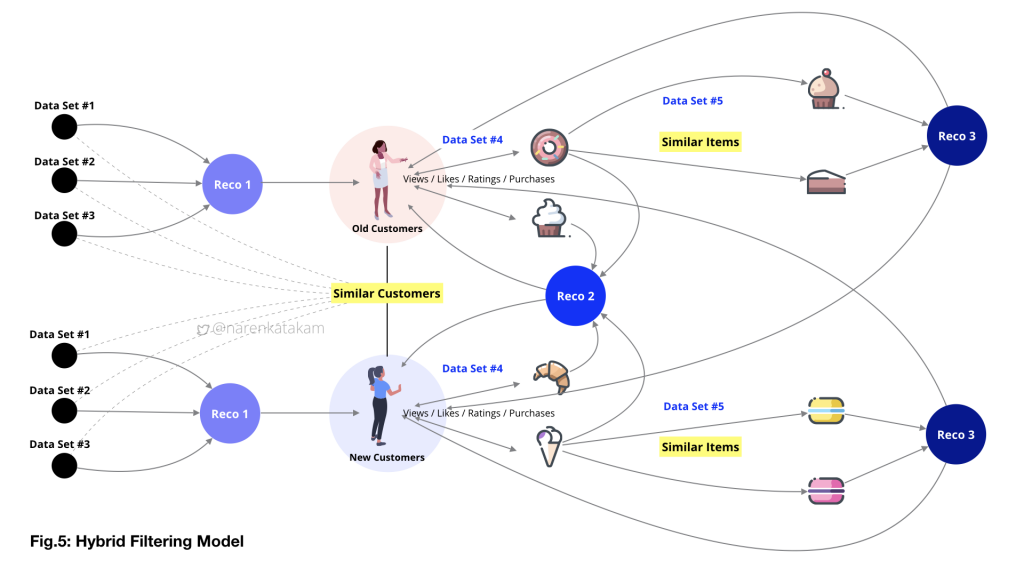
How Can We Improvise Hybrid Filtering Model With Machine Learning?
So far, the above models have been performing pretty well in the product and business world. However, the fundamental assumption around the concept of these models needs a relook. For example, if we take the robust hybrid filtering model, the core assumption here is that — the proposed ‘best’ recommendation to a user is the one that other users with a comparable profile in a comparable state who chose the same or a similar product. In other words, the assumption is that statistically, the recommended product is what the user would’ve chosen with or without the recommendation. The system is picking the best. But, would the user really would’ve chosen the same product without the recommendation? Do recommendations change user behavior or do they merely shortens the path of an inevitable choice? Essentially, the subject of recommendation reduces to statistical analysis of understanding users, products and their relationship. The goal of the system design should be to reduce the information or data that is useless and irrelevant for effective decision making and to stimulate buying action by the user.The question is, how can we improvise? how can we design a system that can learn to understand the nuances of user behavior, take various aspects of business into consideration and then statistically analyze the best recommendation, with the right balance?
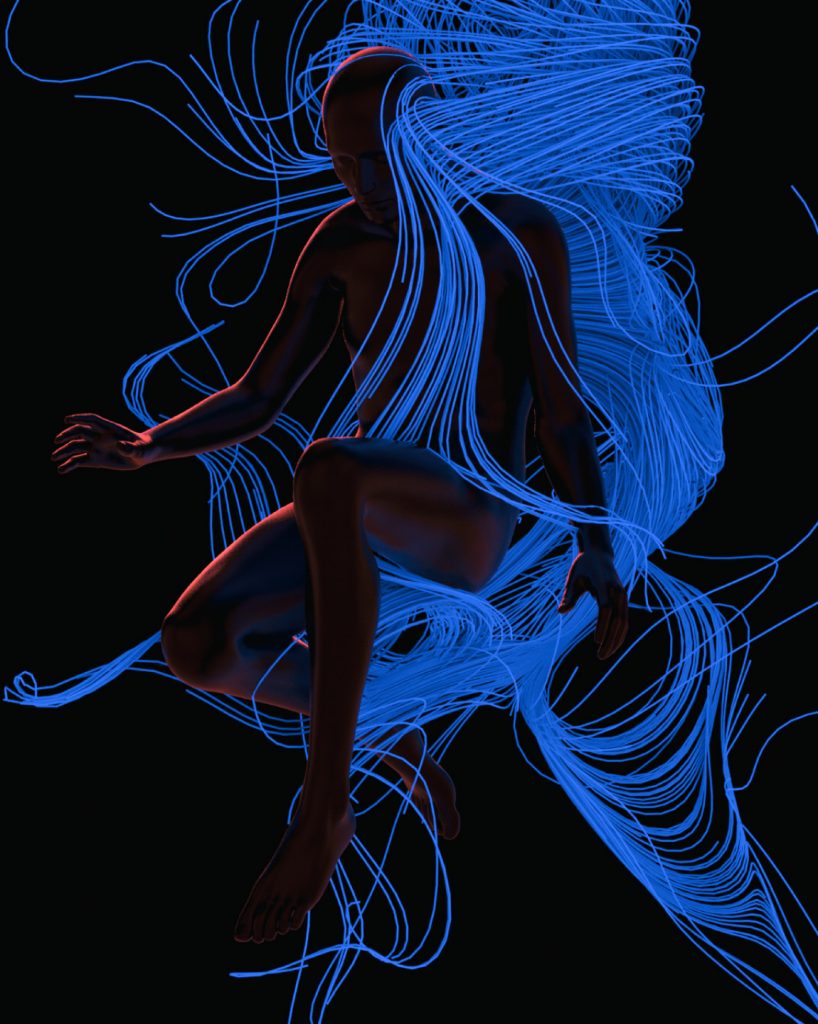
Implicit & Explicit Data Capture At Product Design Level
Since the subject is now boiled down to statistical analysis and relationships and when we talk about modern statistical analysis and techniques to identify patterns from large data sets, machine learning and data mining techniques become an obvious choice. Data is the most essential component of any model and machine learning models thrive on data. However, handling data can be quite complicated, and there are lots of things that can go wrong like, inconclusive data, noise in the data, the readiness of data, bias in data collection and confidence intervals. Data is also extremely crucial to keep the ‘entropy’ of the entire system in check. The ‘purer’ the data-sets and their sub-sets the minimal the entropy of the system will be in the long run.
To generate personalized, specific and tailored recommendations it is essential to understand the users accurately and the first step in understanding them accurately is to collect the right data about them. User data can be collected ‘implicitly’, which is to record user behavior and recognize patterns and ‘explicitly’, which is to collect data by specific inquiry. Below is an example of a mobile app that I have designed. On the left side screen, you will see the implicit approach, where calendar, contacts and location data is captured to understand the user implicitly and identify early patterns. On the right side of the screen, you will see the explicit interrogative approach where information on user’s ‘interests’ is captured in dynamic bubbles moving up from the bottom of the screen, newer bubbles moving up are based on user’s earlier selection (interactive idea here is to gamify a mundane task of interrogation and make it fun for the user to engage and share more information). The goal is to learn from the user reaction about the relevancy of the items to the user and to form a relationship with the content that will follow in the next screens.
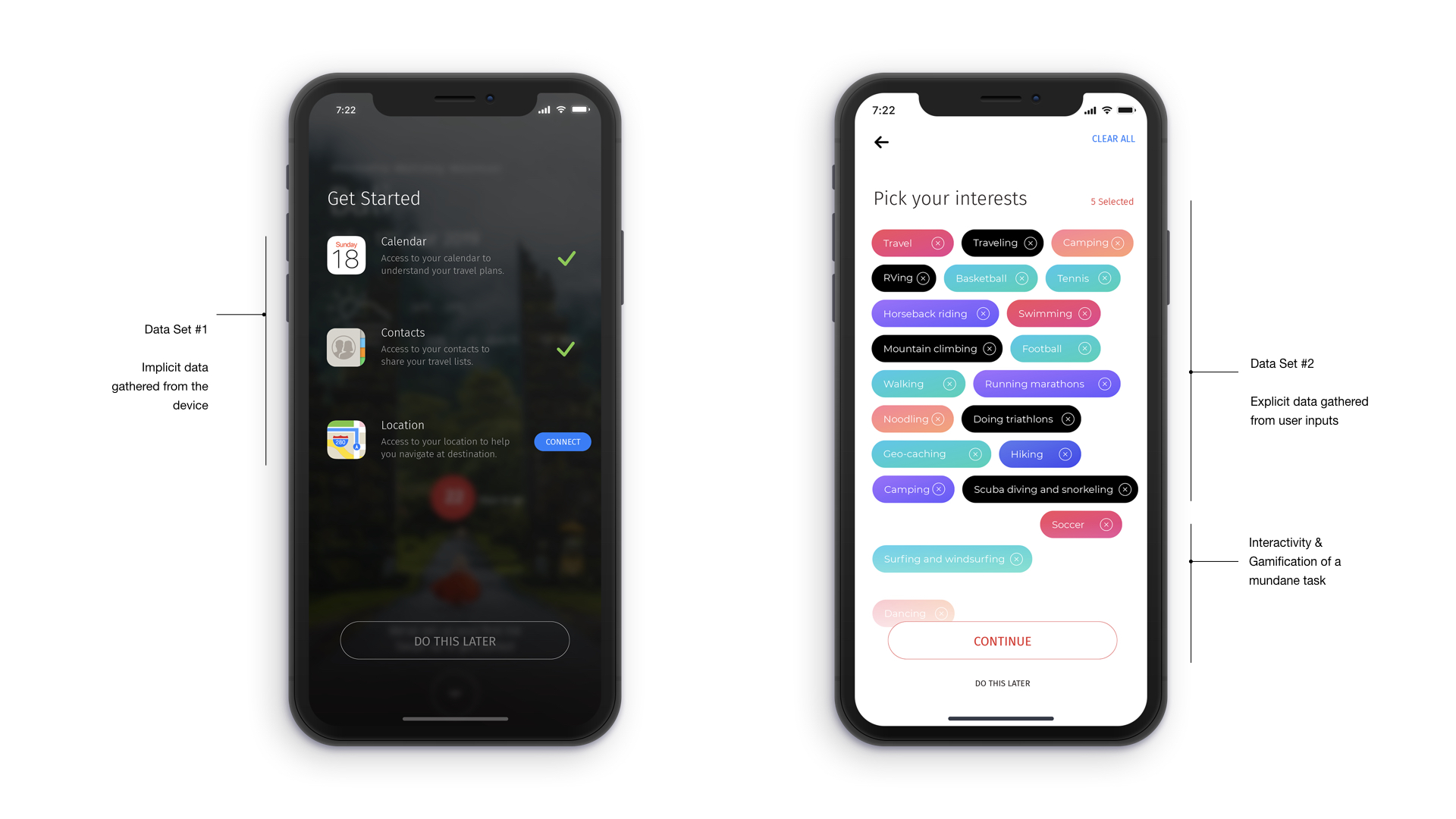
Once we have capture basic requisite data and form data-sets, we should be able to generate the first layer of recommendations (Reco.1) see from Fig.6 below and also possibly identify “similar users” (if we have defined framework in place). The implicit inquiry that started with the onboarding screens can flow into the main content screens, implicit and explicit inquiries can be designed well into the sessions and record user behavior to identify patterns (if any) for future actions. Based on these captures we can form various data-sets like data-set#4 (see from Fig.6 below), this, in turn, forms the input to generate the second layer of recommendations (Reco.2) like a loop.
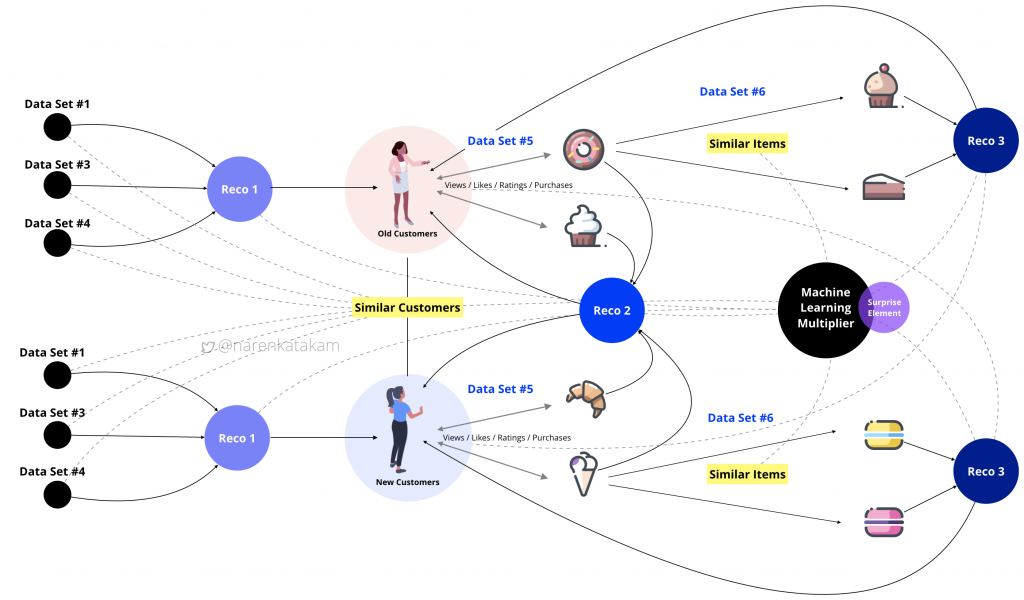
An example of an explicit inquiry can be seen in the screens below, capture and real-time analysis followed by relevant content population. While data set#4 is generated with user’s inputs, data set#5 here is system generated, following an algorithm in identifying similar items and populating content accordingly as the third layer of recommendations (Reco.3).
While Reco.1 is unidimensional, Reco.2 and Reco.3 are multidimensional and based on system learning, learning from similar users and similar items. The goal of the system design here is to increase the effectiveness of data by making it more relevant to stimulate buying action by the user. If the product has special offers as a feature, the complexity of the task even goes a few notches up with frequent varying prices added into the equation (like the screen on the right in the image below).
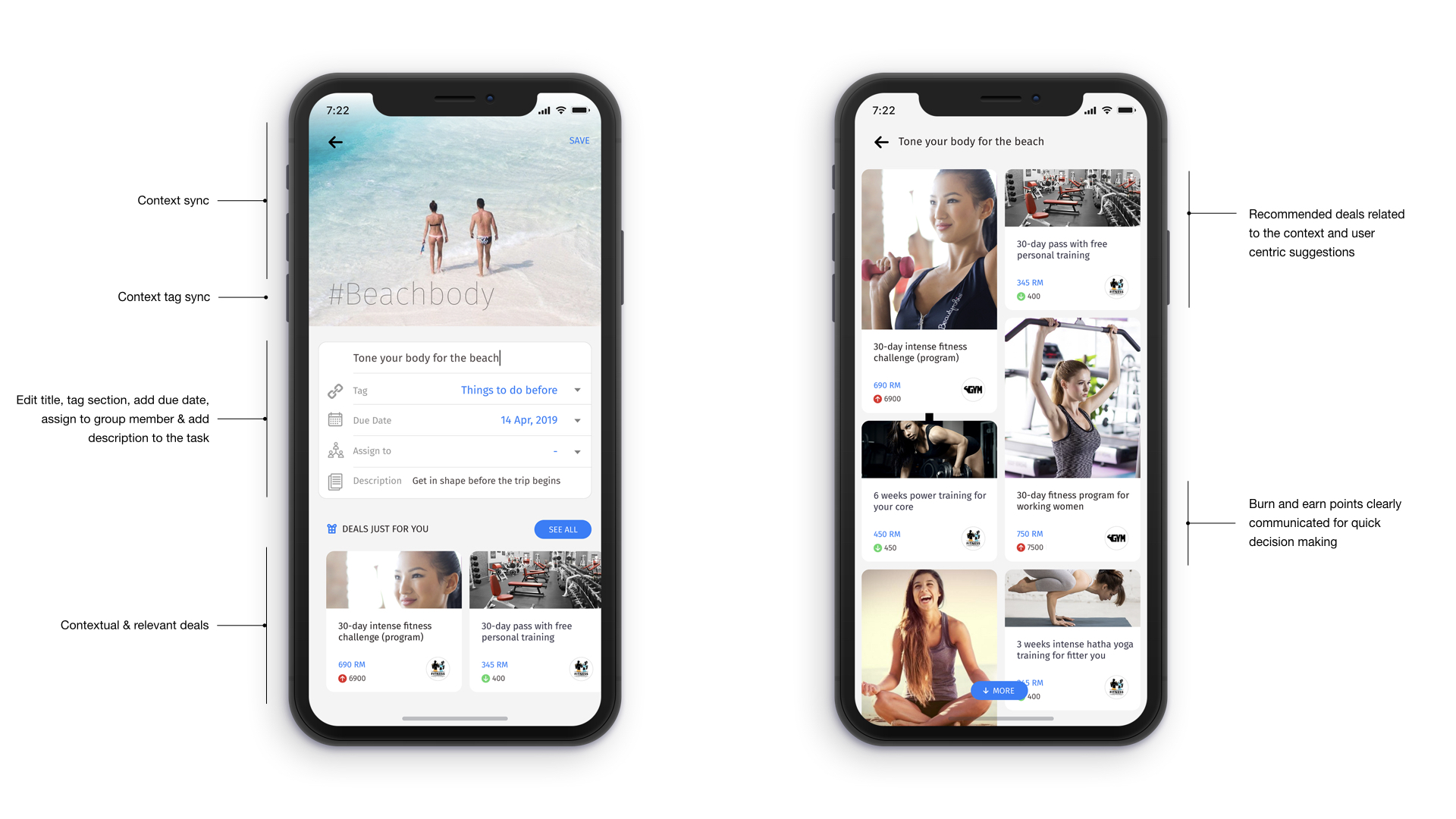
A good recommendation engine should be in a position to learn, adapt and deliver the best recommendation always. This is possible if we can model the engine to analyze the historical data about the user with respect to the item or items the user may be browsing at a given moment using real-time analysis. Real-time analysis can infuse a sense of ‘proactiveness’ to the system that is different from the present ‘reactive’ nature of recommendation engines that are predominantly depended on analysis of just historical data. One of the quick examples for real-time analysis is Google’s Penguin 4.0. Google updated their site ranking algorithm, Penguin 4.0 to real-time (year 2016). What this means is, Penguin’s data is refreshed in real-time, so the changes in page ranking appear much faster than before (year 2016).
A natural conversational interface that allows simple interactions would be extremely valuable in capturing real-time data. The screens below that I’ve designed for this purpose demonstrates exactly that, an evolution of interface design, a concept of a virtual agent backed by an intelligent recommendation engine in the background capable of even sensing user’s emotional state to adapt and suggest the best recommendation that fits right into user’s need, sometimes even before the need arises. The power of prediction goes a long way in demonstrating product value and building trust. It’s more like an intriguing thought experiment. But, surely we’re getting closer every day to such dream states and dreamy product experiences.
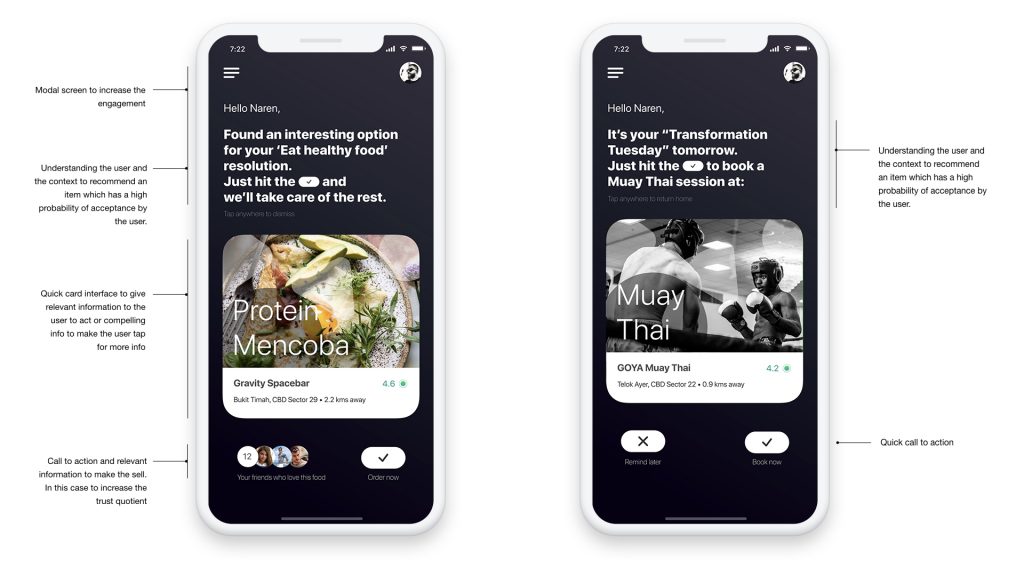
Inclusion Of Systemic Smarts
So the learning of the recommendation engine happens in a step-by-step manner. Goal oriented learning from historical data, learning from real-time interactions, learning from trial and errors in the environment, with rewards and penalties to achieve better accuracies is precisely the task of Reinforcement Learning (RL), one of the most fascinating disciplines of machine learning. Reinforcement learning acts as a multidimensional learning multiplier over this model. According to one of the most standard and comprehensive works on RL, “Reinforcement Learning: An introduction”, by Richard S. Sutton and Andrew G. Barto there are two main elements and three key sub-elements for an RL model. Two elements — an agentand its environment. Three sub-elements, a policy, a reward signal, and a value function.
“Elements: An ‘agent’ is the active decision-making agent and its ‘environment’ is the space or structure within which the agent seeks to achieve a goal despite uncertainty. Sub-elements: A ‘policy’ defines the learning agent’s way of behaving at a given time. A ‘reward signal’ defines the goal in a reinforcement learning problem. A ‘value function’ specifies what is good in the long run.”
Pertaining to our model and context, systemic active decision-making agent (not to be confused with the human decision maker we discussed earlier) and its environment here is the recommendation engine model (from Fig.6), extending from data set#1 to Reco.3. A policy is a mapping of each state and the action. For instance, action to throw Reco.2 as suggestions to a new user based on the state of interactions from a similar old user. The final reward of the model here is the purchase of the product by the user. A more immediate reward is the reaction of the user, like sharing the product with a friend or liking it or bookmarking it or adding to favorites. Value function could be the accumulation of various rewards starting from the first interaction with the system to extrapolating and correcting over the future. The ability to course correct the value function to an incremental value over time can enable the system to add both values to the business and delight to the user in the long run.
Once the engine or system reaches beyond satisfactory levels of accuracy, the next step could be to appropriate the dissimilarity quotient of the items. This can help the system to incorporate the surprise element into recommendations. IMO, getting the surprise element right is finding the holy grail of a recommendation engine.
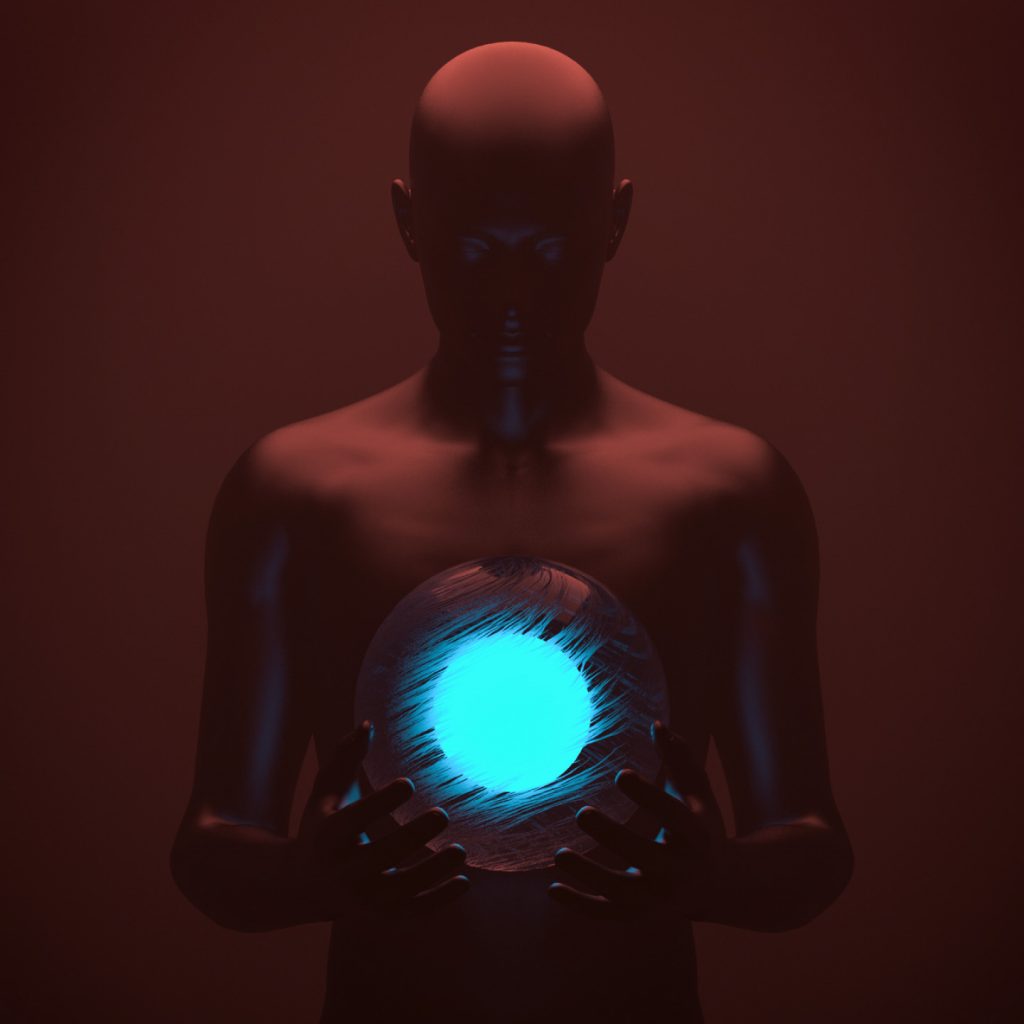
The Holy Grail
The surprise element in this context is the ability of the recommendation engine to recommend a dissimilar item from the known items to a given user in a given situation. The more dissimilar and non-obvious an item is from the known, the more surprise the user can be. The surprise element as a component fits into the content filtering section of the hybrid model because arriving at a dissimilarity quotient is the other side of understanding similar items. The serendipitous nature of discovering an interesting item which user might not have otherwise discovered will boost the delight and help the system to form a deeper bond with the user. This will also help build the ‘trust’ element we spoke of earlier in this post (trust is a fascinating topic to study under game theory). If we have to define ‘serendipity’ as a property of an intelligent recommendation engine, the two most fundamental factors that serendipity is depended is ‘relevancy’ and ‘timing’. From here, we can go deeper into evolving equations for surprise element, serendipity, trust and how it all fits into the overall equation of the gargantuan recommendation engine. Turning a surprise recommendation into a serendipitous occurrence in the context of the recommendation engine is equivalent of the hand of God move (game#4, move#78) in the Go match between AlphaGo & Lee Sedol. Life could be a box of chocolates when an intelligent recommendation engine is handing the box.
Little over a month ago, I was conducting a product innovation workshop; the activities and sessions of that workshop led to an interesting concept of building a recommendation engine at the core as a thin slice of a larger project on top. I started researching over the subject of recommendation engines and how to conceptually design a logically strong structure at the core with an intention to lay a strong foundation for the overall product. I’ve researched customer psychology (decision-making in particular), brick and mortar store walk-ins, various recommendation systems, data science, data mining, AI, machine learning, reinforcement learning, and other related fields. Hundreds of articles, papers, dozens of books and an inspiring Netflix documentary later I didn’t find a single source that connects the high-level systemic design of recommendation engines to grassroots-level interface design. Most of the available material on this subject is from a programming perspective and none from a design or product perspective. So, the idea for this article was born. I have collated most of the references I’ve come across in my research path for this article (list given at the end of this page scroll). If I have missed out mentioning any or if you find an article, a book or a knowledge source that can help me understand this subject better from a systemic ‘design’ and core product ‘logic’ point of view, please feel free to reach me here or on Twitter (my DMs are open on Twitter). Thank you for taking the time to read this article.👏
References:
- http://dataconomy.com/2015/03/an-introduction-to-recommendation-engines/
- https://www.sciencedirect.com/science/article/pii/S095741741830441X?via%3Dihub
- https://www.sciencedirect.com/science/article/pii/S1110866515000341
- https://link.springer.com/article/10.1007/s11257-011-9112-x
- https://www.nytimes.com/2006/10/02/technology/02netflix.html
- https://www.thrillist.com/entertainment/nation/the-netflix-prize
- https://www.amazon.com/Recommender-Systems-Textbook-Charu-Aggarwal/dp/3319296574/
- https://www.amazon.com/Machine-Learning-Recommender-System-Beginners-ebook/dp/B07DWS346Y/
- https://www.amazon.com/Building-Recommender-Systems-Machine-Learning-ebook/dp/B07GCV5JCZ/
- https://www.amazon.com/Machine-Learning-Paradigms-Analytics-Intelligent-ebook/dp/B07F7V67Y5/
- http://arno.uvt.nl/show.cgi?fid=131711
- https://www.wsj.com/articles/models-will-run-the-world-1534716720
- https://www.datasciencecentral.com/profiles/blogs/adapting-an-algorithm-to-real-time-applications
- https://web.stanford.edu/class/psych209/Readings/SuttonBartoIPRLBook2ndEd.pdf
- https://pdfs.semanticscholar.org/e791/6b7daca648de96c93bcc057912d6b1e81b5c.pdf
- https://www.netflix.com/title/80190844
I appreciate, cause I found just what I used to be having a look for.
You have ended my 4 day lengthy hunt! God Bless you man. Have a nice day.
Bye
It抯 onerous to find knowledgeable people on this topic, however you sound like you realize what you抮e talking about! Thanks
Your home is valueble for me. Thanks!?
Youre so cool! I dont suppose Ive read something like this before. So good to seek out anyone with some authentic ideas on this subject. realy thanks for beginning this up. this web site is one thing that’s wanted on the web, somebody with a little bit originality. useful job for bringing something new to the internet!
Thanks a lot for giving everyone remarkably splendid opportunity to check tips from this web site. It is always very ideal and also stuffed with a great time for me personally and my office fellow workers to search the blog at minimum 3 times in 7 days to read through the fresh guides you will have. Not to mention, we are certainly impressed with all the surprising pointers you give. Some two ideas in this posting are rather the simplest we have all ever had.
I intended to send you one bit of word to be able to thank you very much yet again for those superb tricks you have shown on this page. This has been surprisingly open-handed of you to convey easily just what most people could have distributed for an e-book to make some money for themselves, certainly considering the fact that you could have done it if you desired. The concepts as well worked like a easy way to comprehend most people have the same zeal really like my personal own to figure out a lot more regarding this issue. I think there are some more fun occasions up front for individuals that start reading your site.
Very interesting to read you
Good luck to you
I have to voice my admiration for your kind-heartedness giving support to people that need help on this important question. Your special commitment to getting the message up and down appeared to be especially productive and have without exception made ladies like me to realize their dreams. The valuable instruction can mean much a person like me and somewhat more to my colleagues. Warm regards; from all of us.
Do you mind if I quote a few of your articles as long as I provide credit and sources
back to your website? My blog site is in the very same area of
interest as yours and my users would genuinely
benefit from a lot of the information you present here.
Please let me know if this ok with you. Cheers!
Look at my page; Ira
It’s ok.
I am just writing to make you be aware of of the nice discovery my wife’s princess experienced studying your web site. She realized some details, which include how it is like to have a very effective helping character to have many people without difficulty know precisely a number of multifaceted subject areas. You actually did more than my desires. Many thanks for presenting those productive, safe, edifying and even unique tips on the topic to Jane.
Thank you a lot for giving everyone such a brilliant possiblity to read critical reviews from this website. It is often very cool and as well , full of a good time for me personally and my office fellow workers to visit your website nearly 3 times in a week to find out the latest issues you have got. And of course, we’re at all times motivated with your stunning tactics served by you. Selected 4 facts in this post are undoubtedly the most effective we have ever had.
I must show some appreciation to this writer for rescuing me from this type of problem. Just after scouting throughout the the web and seeing techniques which were not helpful, I believed my entire life was well over. Living without the approaches to the issues you’ve resolved by means of your entire guideline is a critical case, as well as the ones that could have in a negative way damaged my career if I hadn’t encountered the blog. Your talents and kindness in touching all the stuff was excellent. I am not sure what I would’ve done if I hadn’t encountered such a stuff like this. I’m able to at this point look forward to my future. Thanks a lot very much for the specialized and effective guide. I will not be reluctant to recommend your blog post to anyone who should get guidelines on this area.
Thank you so much for giving everyone an exceptionally breathtaking chance to check tips from this blog. It’s always very ideal and packed with fun for me personally and my office acquaintances to search your web site not less than three times a week to read the newest issues you have. And of course, I am just always pleased with the exceptional thoughts you serve. Certain 2 tips in this post are ultimately the most beneficial I’ve had.
I simply wanted to say thanks once again. I am not sure the things that I would’ve done in the absence of the information shared by you directly on this area. It was before the intimidating problem for me, however , viewing your specialized form you managed it made me to jump for joy. I am just thankful for the help and thus sincerely hope you realize what a great job that you are getting into teaching most people via a blog. I know that you haven’t met all of us.
My spouse and i have been very excited Louis could do his web research because of the precious recommendations he came across from your very own site. It is now and again perplexing to just find yourself handing out steps which usually some others could have been trying to sell. So we acknowledge we now have the website owner to give thanks to because of that. Most of the explanations you’ve made, the simple web site navigation, the relationships you make it easier to promote – it’s all exceptional, and it is making our son in addition to the family reason why the article is satisfying, which is certainly particularly pressing. Thank you for everything!
I needed to write you one very little note to finally thank you so much again relating to the pleasant concepts you’ve discussed in this case. This has been certainly particularly open-handed with people like you to provide unreservedly just what some people could have made available as an ebook to make some profit for their own end, especially now that you might have done it if you decided. The solutions additionally worked as a easy way to fully grasp that other people online have similar dream just like mine to grasp a good deal more when considering this problem. I know there are millions of more pleasant sessions up front for many who looked over your site.
Thanks so much for providing individuals with such a special chance to read critical reviews from this web site. It’s usually so kind and also jam-packed with a good time for me and my office peers to visit your blog at minimum thrice every week to study the latest guidance you will have. Not to mention, I’m just certainly satisfied concerning the staggering advice you serve. Some 4 facts in this posting are clearly the simplest I have ever had.
Hi to all, how is all, I think every one is
getting more from this web page, and your views are good in support of new visitors.
I really wanted to compose a simple word in order to appreciate you for the magnificent suggestions you are sharing on this website. My time intensive internet search has finally been compensated with excellent know-how to talk about with my best friends. I ‘d admit that most of us website visitors are unequivocally blessed to dwell in a great place with very many perfect people with useful concepts. I feel truly blessed to have used your entire website page and look forward to so many more brilliant minutes reading here. Thanks a lot once again for everything.
It’s an remarkable post designed for all the internet users; they will obtain advantage from it I am sure.
hey there and thank you for your info – I’ve certainly picked up something new from right here.
I did however expertise some technical issues using this web site, since I experienced to reload the
site a lot of times previous to I could get it to load correctly.
I had been wondering if your web host is OK?
Not that I’m complaining, but slow loading instances times will sometimes affect your placement in google and
can damage your high quality score if advertising and marketing with Adwords.
Well I am adding this RSS to my email and can look out for a lot more of your respective fascinating
content. Make sure you update this again very soon.
Attractive section of content. I just stumbled upon your website
and in accession capital to assert that I get actually
enjoyed account your blog posts. Any way I’ll be subscribing to your feeds and even I achievement you access consistently
quickly.
Hi there, I discovered your web site via Google whilst looking for a related topic, your web site came up, it appears to be like good.
I have bookmarked it in my google bookmarks.
Hello there, simply was alert to your weblog thru Google,
and located that it’s really informative.
I’m going to be careful for brussels. I’ll be grateful if you continue this in future.
Many other people will likely be benefited out of
your writing. Cheers!
You can certainly see your skills in the work you write.
The sector hopes for even more passionate writers such as you who aren’t afraid
to say how they believe. At all times follow your
heart.
Magnificent beat ! I would like to apprentice whilst you amend your site, how can i subscribe for a weblog
site? The account aided me a appropriate deal.
I were a little bit acquainted of this your broadcast provided brilliant transparent concept
you’ve got an incredible weblog here! would you like to make some invite posts on my blog?
I’ve been browsing online more than three hours today,
yet I never found any interesting article like yours.
It is pretty worth enough for me. In my opinion, if all web owners and
bloggers made good content as you did, the internet will be much
more useful than ever before.
After looking into a number of the blog articles on your website, I truly appreciate your technique of writing a blog.
I saved it to my bookmark webpage list and will be checking back in the near future.
Please visit my website too and let me know your
opinion.
my web-site … https://www.misterpoll.com/
I blog quite often and I really appreciate your content.
The article has really peaked my interest. I will bookmark your website and keep checking for
new details about once a week. I subscribed to
your RSS feed as well.
Awesome issues here. I’m very happy to look your post.
Thank you a lot and I am looking ahead to contact you.
Will you kindly drop me a e-mail?
This web page is really a stroll-through for all of the data you wanted about this and didn抰 know who to ask. Glimpse here, and you抣l undoubtedly uncover it.
Can I simply say what a reduction to seek out somebody who really knows what theyre speaking about on the internet. You positively know learn how to deliver a problem to light and make it important. Extra people have to read this and perceive this side of the story. I cant consider youre not more popular since you positively have the gift.
Spot on with this write-up, I actually suppose this website wants way more consideration. I抣l most likely be again to read much more, thanks for that info.
Your place is valueble for me. Thanks!?
I抎 should examine with you here. Which is not one thing I often do! I get pleasure from reading a submit that will make individuals think. Additionally, thanks for permitting me to remark!
fantastic issues altogether, you simply received a brand new reader. What might you suggest about your publish that you simply made some days in the past? Any positive?
you have got a fantastic weblog here! would you wish to make some invite posts on my weblog?
Everything is very open with a clear explanation of the issues.
It was really informative. Your site is very helpful. Thanks for sharing!
Pretty element of content. I simply stumbled upon your site and in accession capital to assert that I acquire actually enjoyed account your weblog
posts. Any way I will be subscribing for your augment or even I achievement you access consistently fast.
I’m not sure why but this website is loading very slow for me.
Is anyone else having this problem or is it a problem on my end?
I’ll check back later on and see if the problem still exists.
Hi, I do think this is an excellent site. I stumbledupon it 😉 I may
revisit yet again since i have saved as a favorite it. Money and freedom is the best way
to change, may you be rich and continue to guide others.
Great article. I’m going through a few of these issues as
well..
If you are going for best contents like myself, simply visit this website daily since it presents feature contents,
thanks
great points altogether, you just received a brand new reader.
What would you suggest in regards to your post that you just made a
few days ago? Any certain?
I like the valuable information you provide in your articles.
I’ll bookmark your blog and check again here regularly. I am quite sure I’ll learn many
new stuff right here! Best of luck for the next!
Unquestionably believe that which you said. Your favorite justification appeared to
be on the web the easiest thing to be aware of. I say to you, I certainly get irked while people think about worries
that they plainly don’t know about. You managed to hit
the nail upon the top and defined out the whole thing without having side effect
, people could take a signal. Will likely be back to get more.
Thanks
Hello, for all time i used to check website posts here early in the
daylight, for the reason that i like to gain knowledge of more and more.
It is perfect time to make some plans for the longer
term and it’s time to be happy. I have read this publish
and if I may just I wish to recommend you few interesting issues or suggestions.
Perhaps you can write next articles regarding this article.
I wish to learn more issues approximately it!
My brother recommended I might like this blog. He was totally right.
This post actually made my day. You can not imagine just how much
time I had spent for this info! Thanks!
Pretty nice post. I just stumbled upon your blog and wanted to
say that I’ve really enjoyed browsing your blog posts.
After all I will be subscribing to your feed and I hope you write again very soon!
What’s up it’s me, I am also visiting this site on a regular basis, this web page
is truly fastidious and the visitors are actually sharing nice
thoughts.
Great content! Super high-quality! Keep it up! 🙂
Awesome post! Keep up the great work! 🙂